PI: James (Yichang) Tsai
Co-PI(s):
Institution(s): Georgia Institute of Technology
Abstract
A disproportionally high number of fatal crashes (25%) occur on horizontal curves, even though curves represent only a fraction of the roadway network (5% of highway miles). MUTCD requires various horizontal alignment warning signs to ensure roadway safety on curves. However, current transportation agencies? practices for inventorying existing curve signs are typically a costly manual procedure. This report presents automatic curve sign detection (ACSD) using low-cost mobile devices such as smart phones, machine learning, and crowdsourcing to develop a live curve sign inventory. This inventory can be used to analyze MUTCD curve sign compliance for safety improvements. Phase II of this research project focuses on 1) validating the curve sign computation accuracy, 2) recommending data storage and management alternatives, and 3) recommending a methodology for MUTCD curve sign compliance analysis (MCSCA), and 4) presenting a roadmap for implementing the proposed methodology. Based on tests performed in Phase II, the proposed triangulation method, using the images and GPS data collected using smartphones, can achieve accuracy within 10 meters for most cases. However, some cases can have an error greater than 15 meters. Error cases typically occur when there is a significant change in vertical alignment or a rough roadway surface. Future refinement on the triangulation method that accounts for the vehicle?s pitch angle is recommended. Additionally, data storage and management alternatives and a methodology for MUTCD curve sign compliance analysis are recommended. Finally, a roadmap for implementing the proposed ACSD, live curve sign inventory, and MCSCA is presented.
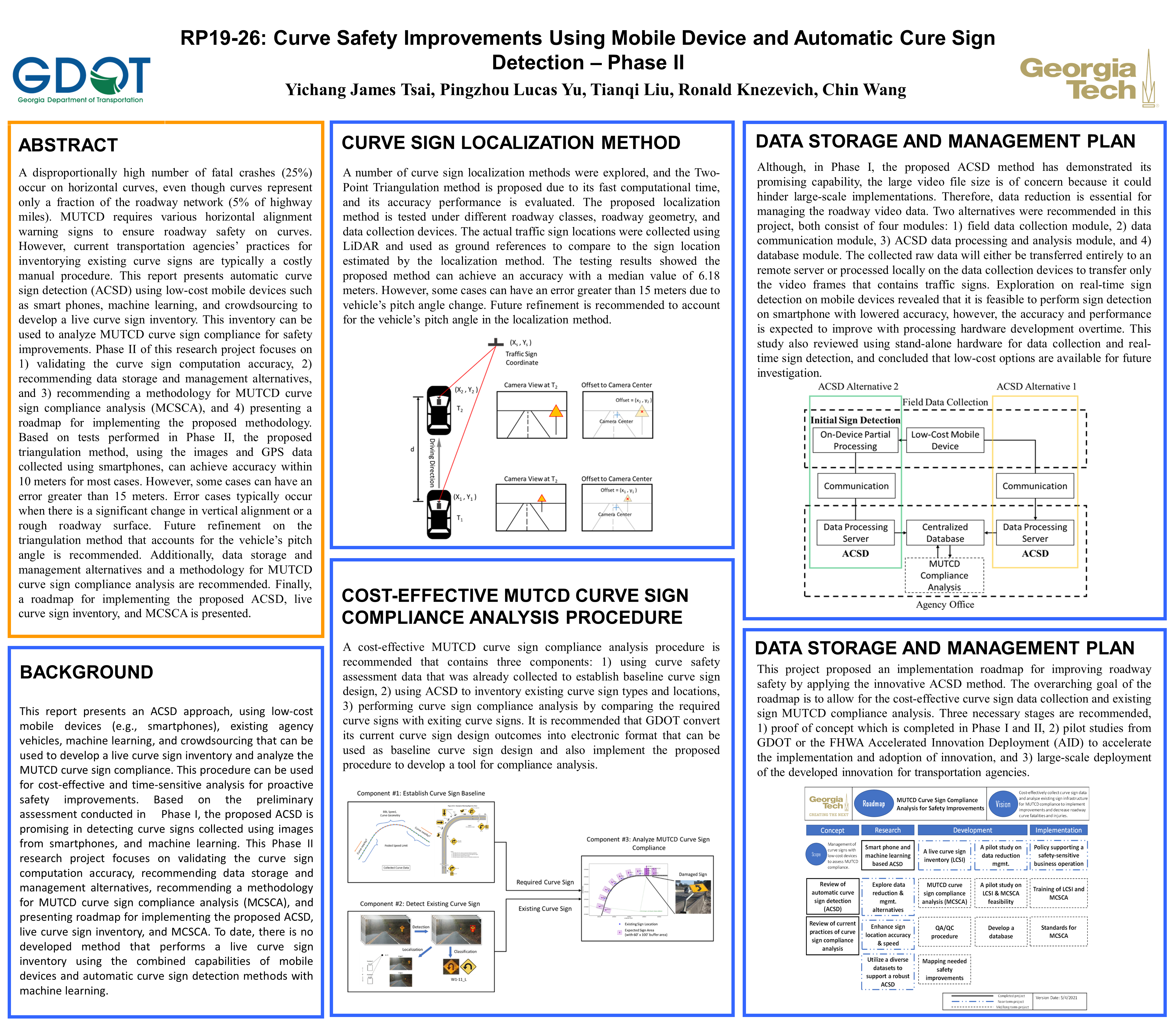
Please comment below with any statements or questions you may have. Also let GTI if you would be interested webinars or presentations on similar topics.