HIGHWAY STORMWATER RUNOFF ON-SITE TREATMENT USING BIOSLOPE WITH NEW MEDIA OF BIOCHAR AMENDED TOPSOILS
PI: George Fu
Co-PI(s):
Institution(s): Georgia Southern University
Abstract
Biochar is typically fabricated from wood biomass, which is readily available and cheaper to obtain in Georgia. This study explored a new media of mixture of biochar and topsoil for bioslope. In this study, four (4) topsoil series (Tifton, Cecil, Pacolet, and Cowarts) were sampled across Georgia, analyzed, and amended with 5, 7, and 10% (weight percent, wt %) biochar to treat highway stormwater runoff through infiltration. Three (3) biochar products from the established manufacturers were selected and screened based on their properties and treatment efficiencies. By utilizing biochar amended topsoil as a new bioslope media, the removal performances exceeded 80% for TSS, total dissolved solids (TDS), total solids (TS), and 60% for oil and grease, ammonia nitrogen, nitrate nitrogen, total Kjeldahl nitrogen (TKN), total nitrogen (TN), and phosphorus with only 5% biochar amendment to the topsoils. For a three (3) yd3 installation volume, 5% biochar amended topsoil was 60% less costly in terms of materials than the current GDOT engineered topsoil for bioslope. Bioslope of biochar amended topsoil will minimize the material cost in construction while providing a green and sustainable alternative compared to the current GDOT bioslope.
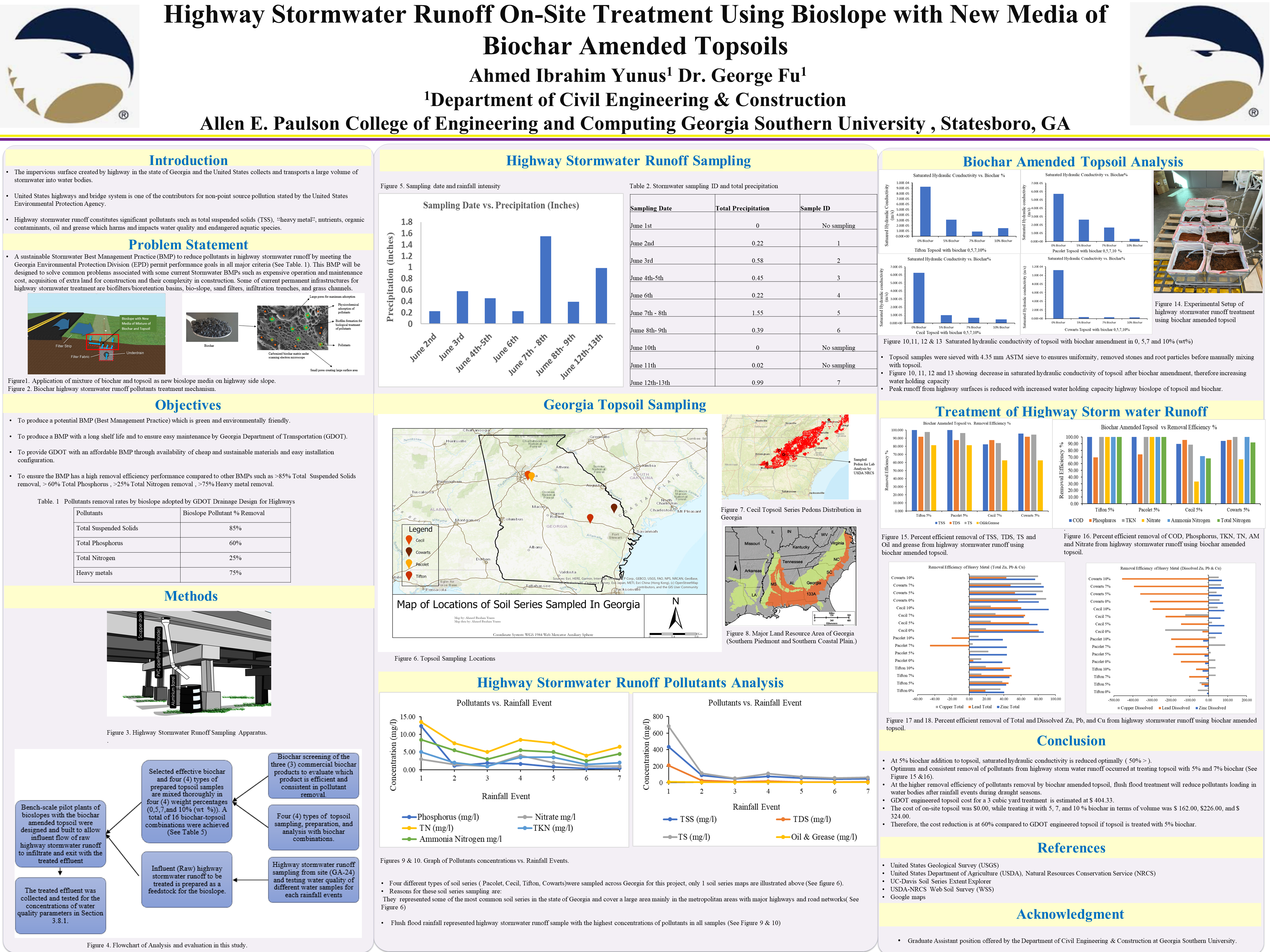
Please comment below with any statements or questions you may have. Also let GTI if you would be interested webinars or presentations on similar topics.