WEIGHT-IN-MOTION (WIM) DATA SYNTHESIS & VALIDATION – DEEP LEARNING MODELS FOR VEHICLE CLASSIFICATION
PI: Mi Geum Chorzepa
Co-PI(s): Jidong J. Yang, Stephan Durham, and Sonny Kim
Institution(s): University of Georgia
Abstract
Sensor-based nonintrusive systems were able to successfully report up to 8 classes of vehicle types while the traditional video-based systems could only distinguish up to 4 classes of vehicle types. Recently, machine-learning methods (Naïve Bayes, K-nearest neighbor classification, random forest, and support vector machine) and deep-learning vision models (CNNS, Faster RCNN, and YOLO models) have been applied for vehicle classification with improved accuracies. However, vehicle classification with the increased number of categories, such as 13 FHWA classes, is still a challenging task. This study investigates a composite model architecture by leveraging the state-of-the-art vision transformer models coupled with a wheel-detection model for complementary feature learning.
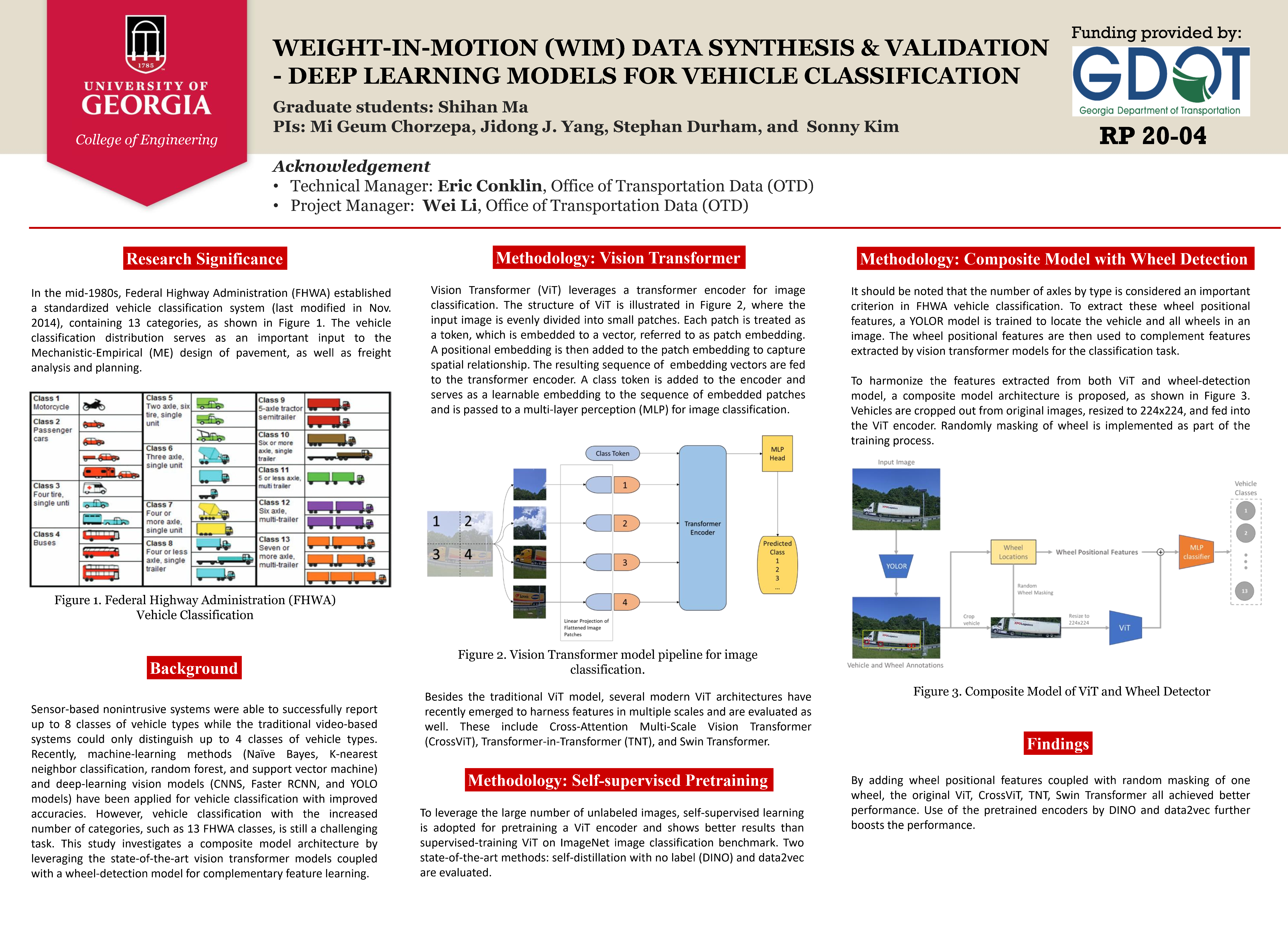
Please comment below with any statements or questions you may have. Also let GTI if you would be interested webinars or presentations on similar topics.